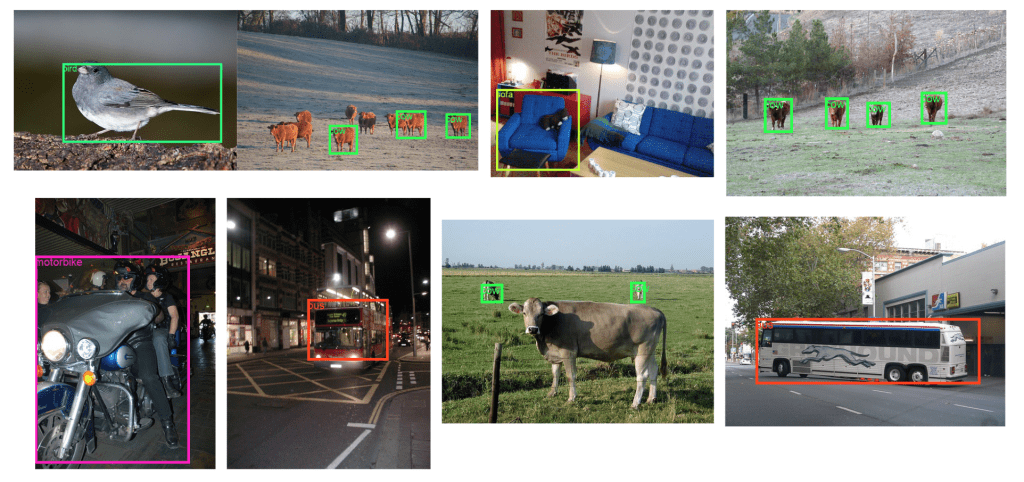
Given its affordability, bounding box annotation is one of the most used labeling methods in computer vision applications. A bounding box can name, measure, and count practically everything. Hence it is used in every business.
- In the medical field, finding aberrant cells in blood smears
- Geospatial: utilizing drones to count the number of animals in a field
- Automobile: spotting vehicles and pedestrians for self-driving automobiles
- Industrial: tally the number of manufactured goods
- Estimating plant size and number in agriculture
- Retail: labeling of already-existing goods on grocery store shelves
Any person could probably do the task of drawing a box around an item with ease.
It is, too. However— Read also – Guest Posting site
Things are a little different when it comes to creating bounding boxes to train your computer vision project ideas models. You need to take care of certain things like:
Check for pixel-perfect tightness.
Bounding boxes’ edges should come into contact with the object’s farthest pixels.
Gaps result in numerous IoU inconsistencies (see below). A flawless model could penalize itself by failing to forecast a region where you omitted data while classifying it.
Pay attention to differences in package sizes.
Your training data should have regular variations in box size. In situations when the same sort of item seems smaller than it often does, your model will perform worse.
Very massive items also frequently perform poorly. A larger pixel footprint in large items influences their relative IoU than a smaller pixel footprint in medium or tiny objects.
Lessen the box overlap
It would be best if you prevented overlap at all costs since bounding box detectors are taught to take box IoU into account.
Objects on a pallet or products on shop shelves, like the wrenches below, are examples of congested groupings where boxes may frequently overlap.
These objects will perform noticeably worse if their labels have overlapping bounding boxes.
As long as two boxes regularly overlap, the model will find connecting the box to the object it contains challenging.
Consider the restrictions on the box size.
The size of the objects your label should be determined by the input size of your model and network downsampling.
Your network architecture’s picture downsampling components may lose information about them if they are too tiny.
Interesting use cases of image bounding box annotation
Self-Driving Vehicles: Autonomous, The most common illustration of the necessity for object detection, is automobiles. In order to determine whether to accelerate, halt, or turn, autonomous vehicles must be able to recognize the numerous things on the road.
Also Read : Exploring Bounding Box for Image Annotation
Autonomous Flying: Drones are the most widely used AI-based models that have been trained using computer vision algorithms for object detection, aerial view monitoring, or tracking the objects like tracking the movement of a cricket bat, tracking an individual in a movie, or object detection and tracking.
Robotics, often known as AI robots: AI-based robots assess visual information in real-time to react correctly and quickly adapt to environmental changes. Robots now possess the capacity to detect and distinguish items in a variety of situations accurately.
Face Detection & Recognition: AI-based cameras employ a computer vision-based face detection and recognition system. The bounding box annotation shows how security systems, AI-based apps, and smartphones learn to recognize faces.
A type of biometric technology called face recognition does far more than detecting the existence of a human face. It sincerely seeks to identify whose face it is by identifying its characteristics. Although the most accurate picture annotation approach for creating these AI models for distinguishing persons with similar appearances is point annotation or critical point annotation.
High-Quality Services for Bounding Box Annotation
You may be acquainted with the saying “garbage in, trash out” if you work in the AI business. Correct. One of the best ways to destroy your next computer vision project is to feed your system erroneous training datasets.
Your algorithm can be thrown off by misaligned image bounding box annotation, which can be challenging to identify and rectify. That is why AI firms frequently decide to hire qualified annotators to handle their picture and video annotation projects.
Anolytics provides access to the appropriate resources, methods, and professional annotators. We provide reasonably priced image- and video-based training datasets that are timely offered for your next machine learning project.